Integration of machine learning and pharmacogenomic biomarkers for predicting response to antidepressant treatment: can computational intelligence be used to augment clinical assessments?
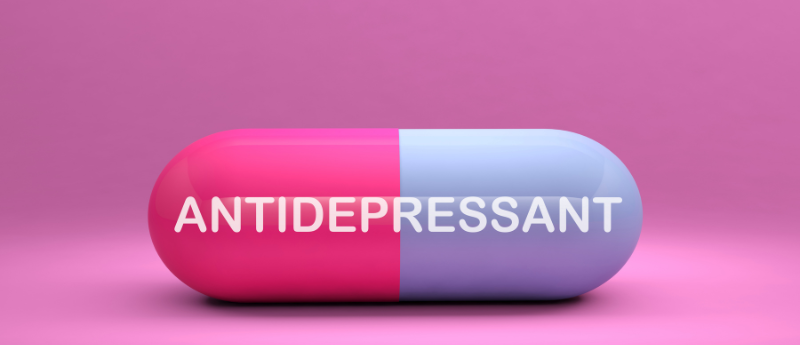
Pharmacogenomics, our partnered journal, has recently published an Editorial discussing the use of machine learning approaches for predicting antidepressant treatment outcomes. Combining information from clinical assessments with pharmacogenomics markers and machine learning tools will enable us to better predict antidepressant treatment therapeutic outcomes.
Major depressive disorder (MDD) is a chronic and relapsing psychiatric illness that affects over 350 million people worldwide and is the leading cause of disability from chronic illness across the globe [1,2]. Fortunately, a large number of first-line treatments are available, making it possible for MDD to be managed in primary care settings as well as mental health specialty clinics [3,4]. However, rates of symptom remission (defined as a near-absence of symptoms) with the most common first-line antidepressants are low – about a third of patients remit after an initial therapeutic trial [5] – and several weeks of treatment are needed before a clear nonresponse profile is established that would enable a change in care [6]. Hence, for many patients with MDD, several therapeutic trials, each lasting several weeks, are needed before a good outcome of treatment with antidepressants is observed [7], thus prolonging disability and suffering in afflicted patients. These challenges highlight the immense value of accurately predicting eventual outcomes of treatment with antidepressants, either before they are initiated or soon after starting them. Such a capability would permit the channeling of depressed patients to specific interventions with the greatest chance of benefit at the soonest possible point in time, given their unique characteristics, in keeping with the goal of precision medicine [8] and with recent calls for a renewed focus on outcome prediction in biological psychiatry research [9].