Pushing the boundaries of ultrasound imaging with deep learning
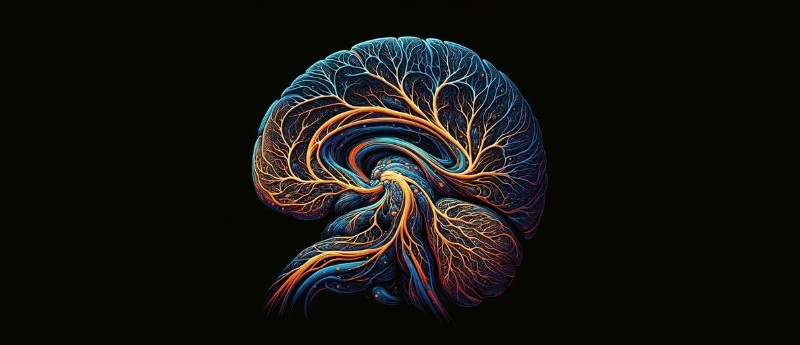
In a landmark study, researchers unveiled a novel approach in medical imaging: Localization with Context Awareness Ultrasound Localization Microscopy (LOCA-ULM). This innovative method leverages deep learning to greatly enhance the precision in detecting microbubbles in blood vessels. Such improvement facilitates superior resolution imaging of the microvasculature deep within tissues.
Ultrasound localization microscopy (ULM) marks a significant leap forward in the ability to image microvascular structures buried deep in tissues. The technique involves tracking microbubbles introduced into the bloodstream, serving as contrast agents. However, traditional ULM methods have faced challenges due to the necessity of spatially isolating these microbubbles. Essentially when these become too densely packed, they are difficult to differentiate from one another. This reduces localization efficiency.
LOCA-ULM markedly improves upon the conventional method by adopting a deep learning-based approach for simulation and localization that adeptly manages dense microbubble environments. The technique not only achieves a remarkable detection accuracy of 97.8% but also significantly lowers the missing rate to 23.8%, outperforming existing methods by substantial margins.
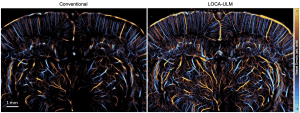
Visual representation of vessel density using the conventional method (left) compared to that developed in the current research (right).
The core innovation of their approach lies in the utilization of generative adversarial networks (GANs) to simulate realistic microbubble signals. This crucial step addresses a significant challenge in the field: the scarcity of real-world training data for deep learning models in ultrasound imaging.
By generating a diverse range of synthetic microbubble signals that closely mimic those found in vivo, the team could train their deep learning model with a high degree of accuracy and realism. This method not only improves the detection accuracy of microbubbles but also drastically reduces the rate at which microbubbles are missed during imaging, outperforming traditional methods and other deep learning-based techniques.
One of the standout accomplishments of LOCA-ULM is its capacity to identify detailed cerebrovascular networks and closely situated microvessels that were previously undetectable with conventional ULM techniques. In experiments involving rat brains, LOCA-ULM has disclosed dense vascular formations and significantly improved the detection sensitivity of functional ULM to blood flow changes, illustrating its potential for cutting-edge brain studies.
Furthermore, LOCA-ULM offers the prospect of shorter imaging durations by facilitating the use of higher concentrations of microbubbles and reducing the computational load, thereby increasing the efficiency of medical imaging practices.
This breakthrough paves the way for non-invasive, high-definition imaging of tiny blood vessels, carrying profound implications for the diagnosis and comprehension of a broad spectrum of vascular diseases, including cancer and neurodegenerative conditions. As the validation and refinement of LOCA-ULM progress, it stands on the brink of transforming the landscape of medical imaging, opening new horizons in medical research and diagnostic procedures.