Decoded Drugs: Introducing our New Weekly Series
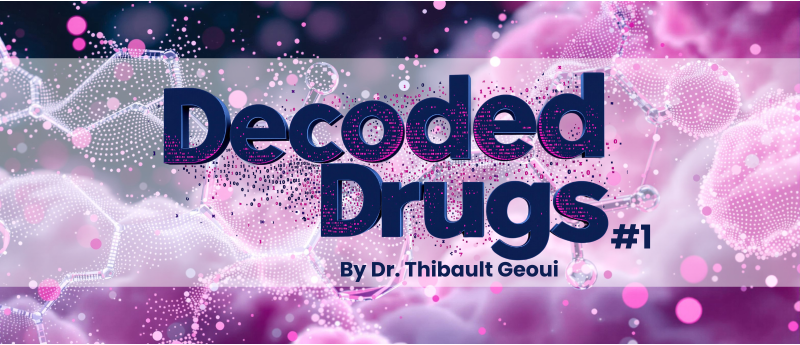
Welcome to “Decoded Drugs”, a weekly column that dissects how AI, data, and technology are reshaping the pharmaceutical industry. Over the coming months, we’ll navigate the hype, celebrate genuine breakthroughs, and confront the thorny questions facing a sector at a crossroads. Can AI reverse decades of declining R&D productivity? Or are we witnessing another cycle of inflated expectations?
I’m Dr. Thibault Geoui, a structural biologist by training, a drug developer by practice, and a technologist by obsession. For 20 years, I’ve worked at the intersection of science and digital innovation, from building data platforms that accelerate target discovery to advising Fortune 500 pharma companies on AI adoption. My goal here is simple: to separate signal from noise in an industry where buzzwords often obscure reality.
Let’s begin with the stakes.
The Stakes: Pharma’s Crisis and AI’s Promise
The pharmaceutical industry is trapped in a paradox. While scientific knowledge has exploded —from Genome Sequencing and CRISPR to large scale automation and sophisticated imaging tools such as cryo-electron microscopy— the cost of developing a single drug has soared to $6 billion1, timelines stretch beyond 10 years, and 95% of candidates fail in clinical trials. This dismal trend, dubbed Eroom’s Law2 (Moore’s Law in reverse), reflects a system buckling under its own complexity.
Enter AI. A recent Boston Consulting Group report claims 67 AI-discovered drugs are now in clinical trials3, with one already approved. Startups like Insilico Medicine boast of slashing discovery timelines from five years to 18 months4. Investors have taken note: TechBio companies have received more than $50 billion since 20155. But beneath the headlines lie critical questions. What defines an “AI-developed drug”? Is this a revolution, or clever rebranding of old science?
What is an “AI-Developed Drug”?
Clarifying the Buzzwords
The term “AI” in pharma often conflates distinct tools: machine learning models that predict protein structures (like AlphaFold), generative algorithms that design novel molecules, and natural language processing systems that mine research papers for overlooked targets. These technologies are not magic; they’re accelerants.
Consider target identification, the first step in drug discovery. AI can analyze genomic databases to pinpoint proteins linked to diseases, for example, UK startup BenevolentAI used machine learning to identify baricitinib, a rheumatoid arthritis drug repurposed for COVID-196. Similarly, generative chemistry platforms like Insilico Medicine’s Chemistry427 or PostEra’s Manifold8 propose molecules optimized for binding affinity and solubility. In 2023, Insilico Medicine used its platform to design a novel USP1 inhibitor for cancer therapy, which advanced to preclinical studies in nine months9. However, these molecules still require synthesis and testing in labs.
The Human-AI Partnership
AI’s greatest value lies in augmenting, not replacing, human expertise. For example, Recursion Pharmaceuticals uses AI to analyze millions of cellular images to identify patterns that human researchers might miss. This approach enables the discovery of novel drug candidates by mapping complex biological relationships. Chris Gibson, CEO of Recursion, has emphasized the synergy between AI and human expertise, stating that their platform allows scientists to focus on interpreting AI-generated insights and designing experiments rather than manually sifting through data10.
The myth of the “fully autonomous AI drug factory” persists, but reality is more pragmatic: AI narrows the haystack, so researchers spend less time searching for needles – another anecdote I heard from Insilico Medicine CEO, Alex Zhavoronkov (paraphrasing) “With GenAI you can remove the haystack and design the perfect needle”
Reality Check: Is this AI or AI-Washing?
Scrutinizing Claims
Of Boston Consulting Group’s 67 “AI-discovered” drugs3, how many were truly born from AI? The line is blurry. Many candidates labelled “AI-driven” involved AI as a minor player – most companies will have a collection of AI models that they use at different stages of Drug R&D, does this qualify the drugs as AI-discovered? True AI-native discovery, where algorithms drive target selection and molecule design and many subsequent steps to drive decision making … remain rare.
Take Insilico Medicine’s INS018_055, a small-molecule inhibitor for TNIK, identified as a potential anti-fibrotic treatment. Touted as an AI-designed drug, INS018_055 was developed through a generative AI pipeline that rapidly progressed from target discovery to preclinical candidate nomination in just 18 months. Unlike traditional discovery methods, AI identified TNIK as a novel therapeutic target by integrating multiomics data, biological network analysis, and AI-powered causal inference.
From there, AI-driven molecular generation produced INS018_055, optimized for both efficacy and drug-like properties. The drug showed promise in preclinical fibrosis models across multiple organs and underwent Phase I trials (NCT05154240, CTR20221542), where it demonstrated safety and tolerability in humans. Yet, like all AI-assisted drug discovery efforts, its ultimate success hinges on how well AI-generated hypotheses hold up against biology’s inherent unpredictability.
While INS018_055 represents a milestone in AI-led drug development, its future in clinical use will depend on whether AI-driven insights translate into meaningful patient outcomes – a test that no algorithm can predict with certainty (yet).
Challenges and Bottlenecks
Four barriers temper AI’s promise:
- Data Garbage In, Garbage Out: AI models rely on high-quality datasets. Yet much biological data is noisy, fragmented, or biased toward well-studied proteins.
- Regulatory Uncertainty: The FDA has approved AI tools for specific tasks (e.g., pathology imaging), but how will it assess drugs where AI influenced multiple R&D stages?
- The Translation Gap: Even perfect algorithms can’t overcome the messy reality of human physiology. A molecule optimized for potency in silicon may fail in vivo due to toxicity or poor bioavailability.
- The Human Factor: Scientists remain skeptical – and in some cases, resistant – toward AI-driven tools. Some fear automation will replace them, while others struggle to trust black-box algorithms over traditional methods. As a result, AI adoption lags, not because of technical limitations, but because changing minds is often harder than changing models.
AI is not a panacea. It’s a tool, one that demands humility.
The TechBio Revolution— Platforms and Players: A Roadmap
The fusion of technology and biology (TechBio) is reshaping drug discovery, clinical trials, and therapeutic design. This series will explore key themes driving this transformation:
1. The TechBio Landscape
- Vertical Integration: How startups like Recursion (with BioHive-2’s supercomputer13) and insilico Medicine (via Life Star’s robotics14) combine AI models, proprietary data, and automated labs (“lab-in-the-loop”) to compress timelines.
- Generative AI’s Promise and Limits: From AlphaFold to foundation models like Phenom-1, we’ll examine whether AI can truly “engineer” biology (as Jensen Huang’s “biology as an engineering discipline” ethos suggests15) or if complexity demands hybrid approaches.
- Big Pharma’s Playbook: Collaboration vs. competition—how Novartis, AstraZeneca, and others are navigating partnerships with TechBio disruptors.
2. Core Challenges
- Biology ≠ Software: Why navigating clinical trials and regulatory hurdles remains a bottleneck for AI-first companies.
- The Black Box Dilemma: Can regulators trust opaque AI models? We’ll dissect the FDA’s stance on explainability16 and bias.
- Data Gaps: The risks of skewed datasets (e.g., genomic underrepresentation) and efforts to build equitable AI tools.
3. The Road Ahead
- Personalized Medicine 2.0: How AI-driven patient stratification (e.g., Tempus18, BioXcel17) could unlock niche therapies.
- Automation at Scale: From cloud labs to robotic platforms like Life Star—will industrialization replace human intuition?
- Collaborative Ecosystems: Breaking silos between computational biologists, clinicians, and regulators, as seen in Moderna’s AI-enhanced mRNA platform19.
What’s Next in the Series?
- Decoding “full-stack” TechBio platforms: Who’s winning the race to integrate AI, data, and lab automation?
- The partnership paradox: Will Big Pharma absorb startups or fuel a new wave of innovation?
- AI in clinical trials: Hype vs. reality in predicting patient outcomes.
- Ethics and economics: Who profits when drug discovery becomes industrialized?
As this column unfolds, we’ll explore these themes in depth, starting next week with a primer on the technologies rewriting drug discovery. The road ahead is fraught with challenges, but for the first time in decades, there’s genuine cause for hope.
About the Author
Dr. Thibault Geoui is a life sciences strategist and advisor specializing in AI-driven drug discovery and digital transformation. With a PhD in structural biology and experience spanning pharma, biotech, and tech, he helps organizations bridge the gap between data, AI, and drug development. Follow Decoded Drugs for weekly insights on how technology is reshaping the future of medicine.