Combatting nicotine addiction with AI
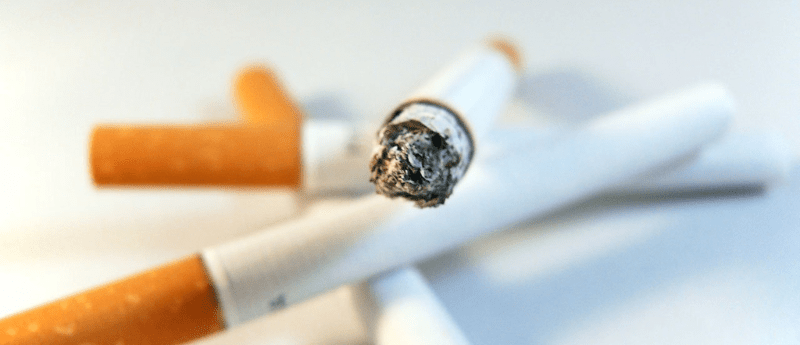
Researchers have analyzed genetic datasets and identified potential drugs for the treatment of nicotine dependence with machine learning.
Drug repurposing is a faster, safer and cheaper alternative to developing and testing novel drugs in therapeutic development. As these agents have already been approved for sufficient safety and efficacy in humans, detailed information is available on their pharmacology, formulation and toxicity. A team of researchers from Penn State College of Medicine (PA, USA) and the University of Minnesota (MN, USA) have utilized machine learning as a drug repurposing strategy to help tackle nicotine dependence.
Cigarette smoking is a risk factor for many human diseases including cancer, respiratory conditions and diabetes. According to the Centers for Disease Control and Prevention, smoking is the leading cause of preventable death.
Smoking behavior is influenced by both environmental and genetic factors – although smoking can be influenced by the social environment and is a learned habit, it is impacted by gene variants that can mean some individuals are more susceptible to nicotine addiction. In fact, it is estimated that genetic factors can explain between 40–70% of variation in smoking initiation. As such, it is important to consider how genetic variation may affect therapeutic effectiveness when discovering drugs to treat nicotine dependence.
In a recent article published in Nature Genetics, the team developed a novel machine learning method that analyzed genetic datasets from more than 1.3 million individuals to identify potential drugs for treatment of nicotine addiction. The datasets also detailed information about self-reported smoking behaviors.
The team discovered over 400 genes that were associated with smoking behaviors. These included genes for nicotine receptor production and dopamine signaling. Using machine learning, the biological pathways for each gene were identified and analyzed to decipher which approved medications could alter existing addiction-associated pathways.
Overall, the model identified at least eight drugs with repurposing potential that shared molecular pathways associated with smoking behaviors and could help individuals quit smoking. These included some medications that are already being assessed in clinical trials, and currently approved medications that are used to relieve coughs caused by the common cold or flu, such as dextromethorphan, and treat Alzheimer’s disease, such as galantamine.
However, the team cautioned some study limitations. The majority of genetic data analyzed by the machine learning model was from individuals with European ancestry, with only a small minority representing individuals with African, Asian or American ancestry. The team stressed the importance of creating representative databases from individuals with varied and diverse ancestries.
“This will only improve the accuracy with which machine learning models can identify individuals at risk for drug misuse and determine potential biological pathways that can be targeted for helpful treatments,” commented study author Bibo Jiang.
Co-author Dajiang Liu, concluded: “Re-purposing drugs using big biomedical data and machine learning methods can save money, time and resources. Some of the drugs we identified are already being tested in clinical trials for their ability to help smokers quit, but there are still other possible candidates that could be explored in future research.”