Augmented intelligence to predict 30-day mortality in patients with cancer
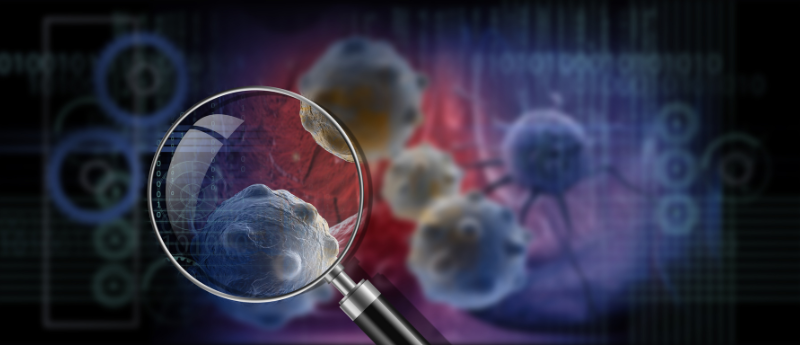
Future Oncology, our partnered journal, has recently published a Short Communication article exploring the use of an augmented intelligence tool to aid cancer risk prediction, enabling clinicians to diagnose and treat patients more quickly.
Abstract
Aim: An augmented intelligence tool to predict short-term mortality risk among patients with cancer could help identify those in need of actionable interventions or palliative care services. Patients & methods: An algorithm to predict 30-day mortality risk was developed using socioeconomic and clinical data from patients in a large community hematology/oncology practice. Patients were scored weekly; algorithm performance was assessed using dates of death in patients’ electronic health records. Results: For patients scored as highest risk for 30-day mortality, the event rate was 4.9% (vs 0.7% in patients scored as low risk; a 7.4-times greater risk). Conclusion: The development and validation of a decision tool to accurately identify patients with cancer who are at risk for short-term mortality is feasible.
In recent years, artificial intelligence (AI), the capability for machines to mimic intelligent human behavior, and its application in machine learning (ML), which allows systems to learn from data and experience without explicit programming, have demonstrated their ability to improve healthcare delivery in a myriad of ways. AI and augmented intelligence (the use of AI to aid rather than replace human decision-making) have found many applications in oncology, from improved accuracy in diagnosis through radiographic image analysis to the ability to predict cancer treatment toxicity [1,2]. Despite these advances, the application of AI and ML to identify patients at risk for short-term mortality who could benefit from either an intervention to modify actionable clinical factors or a referral to palliative care services has received little attention.
While cancer accounts for a significant proportion of deaths in the USA each year, many patients with a diagnosis of cancer die of non-cancer causes [3]. Cancer treatment itself is associated with complications that can be life threatening, including tumor lysis syndrome, febrile neutropenia and sepsis [4–6]. In addition to the risks to individual patients, emergency department visits and hospitalizations related to these complications also carry a considerable financial burden [7]. The ability to proactively identify these patients could facilitate early intervention and resolution of reversible complications.
For patients with cancer who are approaching end of life (EoL), early palliative and hospice care referrals result in improved quality of life and symptom management [8,9]. In contrast, aggressive, life-sustaining EoL care can conflict with patient preference and result in lower quality of life, family perceptions of poorer quality of care, and greater regret about treatment decisions [10]. Earlier referral also represents an opportunity to transform cancer care by reducing the potential for unnecessary, toxic and expensive treatments at EoL. The American Society of Clinical Oncology clinical practice guidelines recommend that palliative care be integrated into standard oncology care for patients with advanced cancer [11]. Additionally, the proportion of patients who died from cancer who received chemotherapy in the last 14 days of life, and the proportion who died from cancer who were admitted to hospice for fewer than 3 days, are quality measures endorsed by the National Quality Forum [12]. Many physicians delay advance care planning and EoL conversations until patients are in the terminal phase of life [13]. The ability of physicians to predict mortality varies greatly and has been demonstrated to be largely inaccurate [14–16]. Existing mortality risk models often only include clinical factors already known to clinicians (e.g., Eastern Co-operative Oncology Group performance status, stage, cancer type and number of comorbidities) and identify patients whom clinicians are already aware of as being at risk [17,18]. However, various sociodemographic and geographic factors can also impact outcomes of patients with cancer beyond clinical factors. The impact of such nonclinical factors upon mortality in patients with cancer is an area of active research.
The aim of this study is to demonstrate that a commercially available AI tool can provide clinicians with insight into which patients with cancer are at the highest risk of mortality in the next 30 days. We developed and validated a ML model at a large community oncology practice in the Pacific Northwest of the USA utilizing clinical, environmental and behavioral data and information on social determinants of health. An objective decision support tool could assist providers in identifying patients who would benefit from a goals of care assessment and palliative and/or hospice consultation.