AI tool can accurately identify ‘hidden’ concussion in athletes
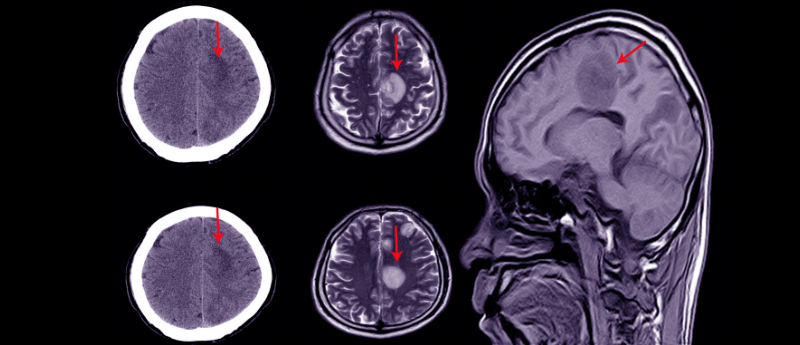
AI can now diagnose concussion in college athletes based on tiny ‘hidden’ structural changes in MRI brain scans.
Concussion and head injuries are extremely common in athletes, particularly in high-contact sports such as football, soccer and boxing. Each year in the US alone, there are around 3.8 million sports-related concussions. In fact, the Center for Disease Control estimates that approximately 5–10% of athletes will have a concussion in any given sports season.
Symptoms of concussion usually disappear within several days, and typically do not extend past several months. However, many injuries go unreported or are poorly managed, which can lead to insufficient recovery and long-term consequences. Repeated head injuries, particularly if the previous concussion has not yet healed, are linked to a range of symptoms in athletes, such as memory loss, sleep disturbances and neurological conditions including chronic traumatic encephalopathy and Alzheimer’s disease.
Unfortunately, most functional and structural brain injuries from concussions are difficult to detect with CT imaging scans unless they are severe. Consequently, it is difficult to accurately diagnose concussion and pick up on tiny structural alterations in the brain.
Researchers from the Department of Radiology at NYU Grossman School of Medicine (NY, USA) have now developed an AI tool that could detect this microscopic brain damage in college athletes.
Utilizing machine learning to analyze hundreds of brain MRI scans of student athletes, the program was able to distinguish between the brains of 36 contact sport athletes (such as football) and the brains of 45 non-contact sport athletes (such as track and field). The program clearly associated repeated head trauma with miniscule structural brain differences in contact sport athletes who had not been diagnosed with concussion.
“Since we expect these groups to have similar brain structure, these results suggest that there may be a risk in choosing one sport over another,” explained Yvonne Lui, a professor in the Department of Radiology at NYU Langone Health.
The machine learning model was trained with previous data from the MRI scans of almost 100 male athletes, from both contact and non-contact sports, who were not diagnosed with a concussion during the model training period. The researchers scored the most useful MRI metrics for detecting damage: mean diffusivity (how easily water can travel through the brain) and mean kurtosis (a marker for tissue heterogeneity that gives important microstructural information). This information was used to optimize the model and improve the concussion diagnosis accuracy.
“Our results highlight the power of artificial intelligence to help us see things that we could not see before, particularly ‘invisible injuries’ that do not show up on conventional MRI scans,” declared study lead author Junbo Chen, a doctoral candidate at NYU Tandon School of Engineering (NY, USA). “This method may provide an important diagnostic tool not only for concussion, but also for detecting the damage that stems from subtler and more frequent head impacts.”
The team aim to further their research and expand the applications of this AI tool for analyzing head injuries in female athletes as well. If we can create highly accurate and sensitive diagnostic tools that can detect tiny ‘hidden’ structural changes in the brain, this may enable us to improve our understanding of how these changes may relate to brain injury and disease.