AI Predicts the Onset of Parkinson’s and Dementia Using Sleep Video Analysis
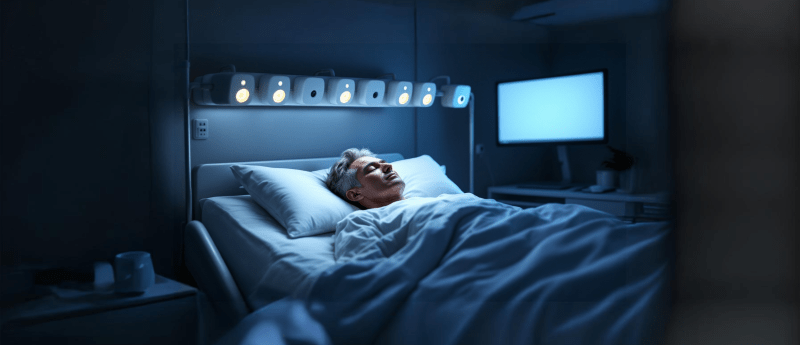
A group of scientists in Mount Sinai (Egypt) have developed a machine-learning tool to diagnose a sleep disorder predictive of both Parkinson’s disease and dementia. The system analyzes standard video footage collected in sleep clinics, potentially allowing for earlier detection and intervention strategies for neurodegenerative diseases.
Sleep Problems Could Signpost Serious Health Concerns Down the Line
Rapid Eye Movement (REM) sleep behavior disorder (RBM) is a sleep condition that affects approximately 40–100 million people.
It is characterized by a loss of normal muscle atonia that occurs during REM sleep cycles, causing afflicted individuals to physically act their dreams whilst asleep. This ranges from minor hand gestures to violent thrashing, kicking or punching.
RBD is a strong predictor of Parkinson’s disease (PD) and dementia, two neurodegenerative diseases caused by synucleinopathy . In fact, studies show that around 97% of people with RBD will develop PD, dementia or multiple system atrophy within 14 years of diagnosis.
Diagnosing RBD early on enables clinicians and patients to develop risk mitigation plans, years ahead of the onset of neurodegeneration. This is particularly important because, in cases of neurodegeneration, lost brain cells cannot be replaced. While the disease cannot be cured, the best possible outcome is to halt its progression.
However, this is easier said than done.
The Challenges of Diagnosing RBD
Diagnosis of RBD requires patients to undergo a sleep study or video-polysomnogram. During this, a range of data is collected about muscle activity, eye movement, and sleep stages. While effective in collecting necessary diagnostic data, it necessitates access to specialized facilities and involves an overnight stay for the patient.
Additionally, staff must trawl through the video footage and data to establish indicators of diagnosis. This manual process is not only time-consuming but introduces subjective bias from human interpretation.
AI Could Carve a Path to Better Diagnose RBD
So, what can be done to help identify more RBD cases?
A recent study, published in Annals of Neurology, highlights the possibility of using AI to analyze video-polysomnograms and identify sleep behaviors associated with RBD, assisting doctors in making fast, accurate, and consistent diagnoses.
Unlike previous AI systems reliant on 3D cameras, which are not standard in clinics, researchers at Mount Sinai designed an AI model to analyze footage from widely used 2D cameras. By incorporating two new features into the model’s analysis, namely movement velocity and magnitude, to the already existing ratio and rate of movement, they significantly enhanced its accuracy.
The team also compared the AI’s performance when evaluating immobility patterns, finding that a high accuracy rating of 92% was achieved when only short durations of immobility (lasting 0.1–2 seconds) were considered.
Unpacking the AI Toolkit
The study outlines a type of model called a nonlinear multilayer perceptron (MLP), a type of feedforward neural network used forsupervised learning tasks, in this instance, for classifying cases as RBD-positive or RBD-negative.
MLP models contain multiple layers that extract patterns in data, the following are the steps involved:
- A linear layer applies a straight transformation to an input, which allows the model to learn the importance of each feature and assign ‘weights’ to them.
- A rectified linear unit (ReLU) activation function is applied, introducing nonlinearity into the network, and enabling the AI to capture more intricate patterns in the data.
- This architecture allows the AI system to capture and extract important sleep behaviors from video footage captured by 2D cameras, enabling accurate and rapid RBD prediction using technology that is already available in sleep clinics (2D cameras).
Important features of ReLU functions:
Negative values (like negative voltages or shifts in oscillating data) in ReLU functions are set to zero, which ‘switches off’ some neurons in the network. As a result, the number of active neurons is reduced, meaning that certain noisy features of the data are ignored. This helps to mitigate overfitting, a common problem with deep neural networks.
In this article, the authors also propose an added L2 regularization component, which further dampens the problem of overfitting by penalizing the model when the calculated importance (weights) of different features become too large.
Here, the bigger the weight, the more importance the model will assign to a particular feature of the data so that errors in the data might have more influence over the AI’s predictions. L2 regularization helps to overcome this issue.
The Bigger Picture
This study is a prime example of how AI can be used to bilaterally improve patient care and healthcare workflows. The findings have the potential to guide the future diagnosis and management of RBD, highlighting how AI can offer a standardized way of identifying RBD without doctors having to scrutinize hours of video footage.
Automated detection of RBD could reduce the number of missed cases and, crucially, flag patients who are at high risk of developing severe neurodegenerative diseases, potentially a decade in advance. Signposting these individuals would allow patients and doctors to prepare; treatment plans could be put in place and started early, which could help slow disease progression more effectively. This could dramatically improve the quality of life and clinical outcomes for these patients.